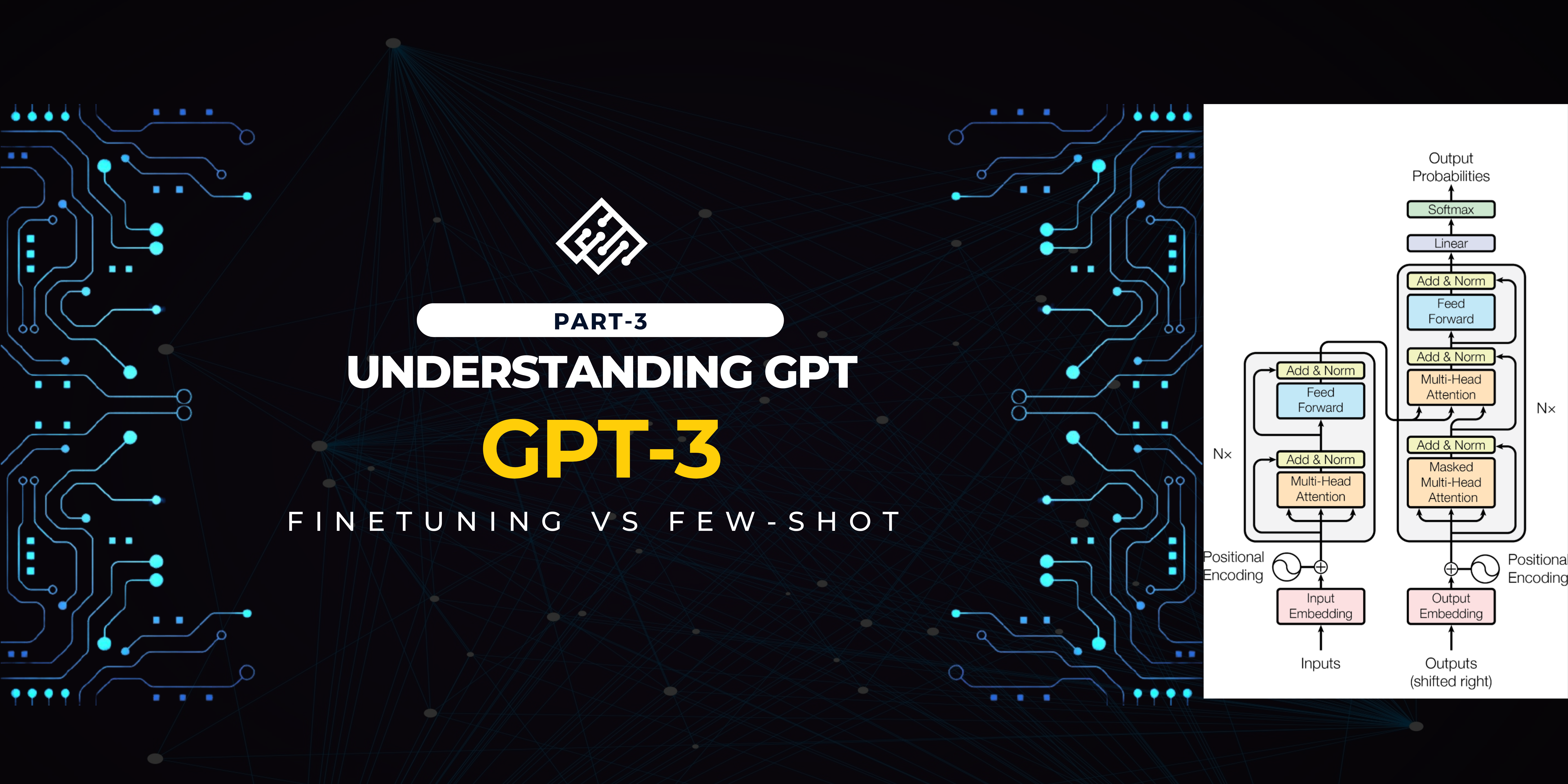
Understanding GPT 1, 2 and 3
Introduction The goal of this series of posts, is to form foundational knowledge that helps us understanding modern state-of-the-art LLM models, and gain a comprehensive understanding of GPT via reading the seminal papers themselves. In my previous post, I covered transformers via the original paper “Attention is all you need” that brought the innovation that made all this progress possible. This post will focus on GPT-3 and its predecessors GPT-1 and 2....